The popularity of web-based platforms such as social networks and booking platforms has led to an unprecedented surge of users. For instance, social networks like Facebook and Twitter have billions of users conducting various activities on their platforms. Thus, this has given the platforms access to massive user-related datasets that can be mined for various supervised and unsupervised user-based tasks. However, learning user preferences is not a straightforward task due to various known challenges, such as data sparsity and the dynamic behavioral patterns of users. Nicholas’ research focuses on developing personalized recommender systems with real-world impact. For instance, successive preferences can be learned from users’ historically visited location sequence to predict where they are going next and provide meaningful recommendations on the platforms. Therefore, he proposed an explore-exploit Spatial-Temporal-Preference User Dimensional Graph Attention Network (STP-UDGAT) model to learn successive preferences of users in multiple domains of Location-Based Social Networks (LBSN), transportation, and counter-terrorism. Further, to better learn users’ dynamic preferences in the transportation domain and provide origin-aware recommendations, he proposed the Spatial-Temporal Origin-Destination Personalized Preference Attention (STOD-PPA) encoder-decoder model. The encoder-decoder framework allows dynamic preferences of each user to be learned and best provide personalized recommendations on their next destinations. Click on the video below to view a presentation on the research project! |
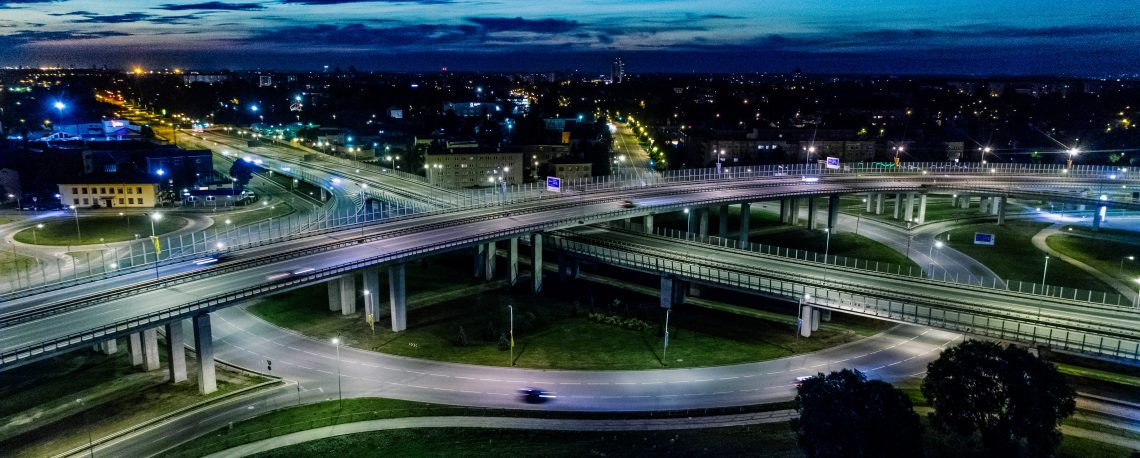