The rapid progress of urbanization has modernized many people’s lives, and inevitably engendered big issues, such as traffic congestion, energy consumption, and pollution. Recently, researchers have tackled these issues by analyzing human mobility data that has been generated in cities, e.g., GPS trajectories, social media posts, and phone records. The key challenge in human mobility analytics is the complexity and diversity in both spatial and temporal domains. Having benefitted from such big data, spatio-temporal deep neural networks have become the dominant class for understanding human mobility and triggered various applications to human mobility. In this study, Yuxuan presents systematic analytics on large-scale human mobility data by stratifying the view into three representation levels (i.e., trajectory level, road level, and region level), each with the corresponding analytic task to be addressed. At the trajectory level, he models the instantaneous change of variables in GPS traces by spatio-temporal neural ordinary differential equations for downstream tasks, such as transportation mode detection and next location prediction. At the road level, he integrates deep spatio-temporal neural networks with physical laws for accurate and interpretable traffic flow forecasting over road networks. At the region level, he jointly considers the spatial, temporal, and external relations to forecast the fine-grained crowd flow data. Extensive experiments on real-world datasets have demonstrated the effectiveness of his approaches. Furthermore, several cloud-based systems have been deployed to show their practicality. Click on the video below to view a presentation on the research project! |
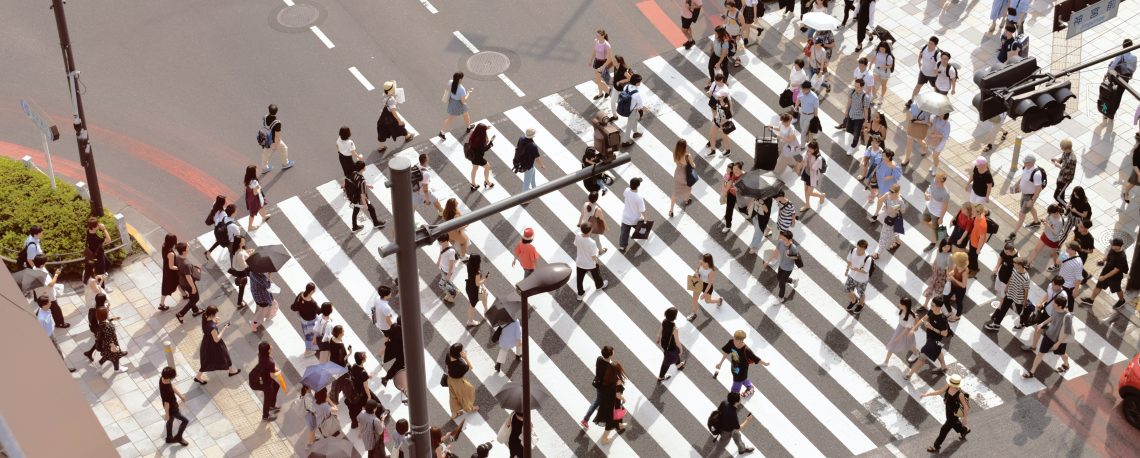