Graph data represents connected entities and their relationships, which appear in many application domains, such as biology, social networks, road maps, the web, communication networks and finance etc. There has been a noticeable increase in the prevalence of work on graph data both in research and in practice, evidenced by the surge in the number of different commercial and research software for managing and processing graphs. In recent years, artificial neural networks have won numerous contests in machine learning on the regular data, such as images, text and speech. However, how to develop effective neural networks for the irregular graph data is still under exploration. Hence, Yiwei’s research aims to develop advanced graph neural network algorithms, as it allows machines to automatically mine and utilize the rich knowledge in the ubiquitous graph data, instead of relying on the efforts of human experts, which is time-consuming and expensive. To this end, his research focuses on developing effective, efficient, and neural network algorithms for the real-world graph data. His contributions include a new set of methods for the challenging tasks on the graph data such as node classification, link prediction, graph classification etc. Click on the video below to view a presentation on the research project! |
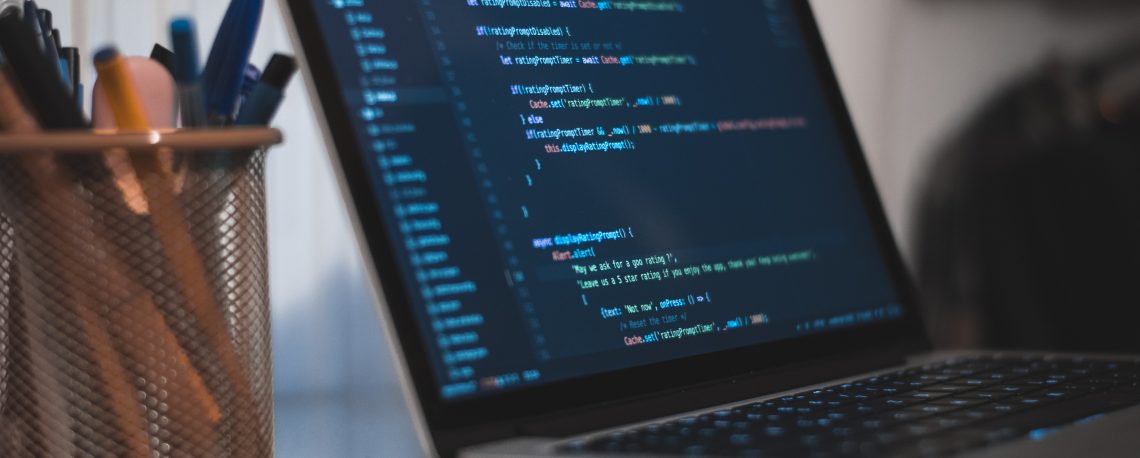