The rise in freight-related activities in urban centres has been a pressing concern for city authorities and policymakers due to their potential to result in issues related to traffic congestion and other negative externalities. Unfortunately, research progress in urban freight studies has been impeded by the significant challenges faced during data acquisition, with current data collection approaches heavily reliant on traditional surveying techniques. This limitation has led to data collection efforts that are time-consuming, labour-intensive, and expensive but also resulted in the collection of sparse and incomplete data, which provides limited insights about the urban freight activities conducted. Raymond’s overall research objective involves developing and applying novel machine learning algorithms to resolve the prevalent data issues commonly encountered in real-world urban freight datasets and gaining valuable insights to support decision-making and urban planning. Some of his past works involve developing a novel imputation algorithm to overcome the incomplete data observed in a  parking and observation survey conducted at nine urban retail malls in Singapore. The imputed data is subsequently used to develop a parking duration model to understand the factors influencing vehicle dwell time. Other works involve proposing an end-to-end point of interest conflation framework to address the data sparsity issues observed in existing geospatial datasets to produce a more comprehensive and enriched dataset for future research use. Lastly, one of his most recent works attempts to apply active learning during the conduct of urban freight surveys to significantly reduce the cost of data collection while ensuring that the data collected is representative of the population. Click on the video below to view a presentation on the research project! |
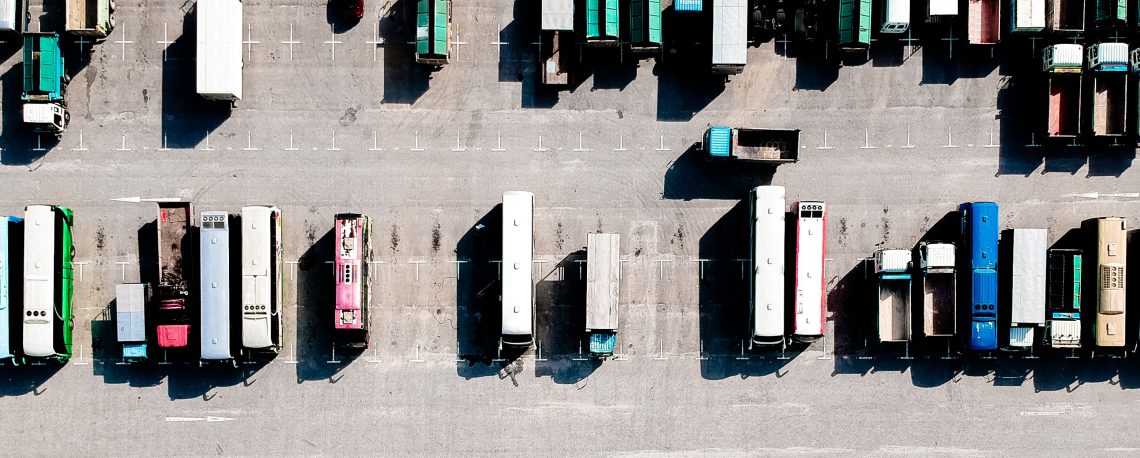