Image restoration is a crucial area of research in computer vision that involves taking a degraded or noisy image as input and producing an estimate of its clear or original image. With the rise of smartphones, low-light image enhancement, a type of image restoration task, is becoming increasingly important. Due to the small size of CMOS in mobile devices, a limited number of incoming photons result in intense noise when captured in low-light conditions. Unlike traditional denoising tasks where the noise is typically synthetic, the noise distribution in low-light images is more challenging due to its spatial dependence. Thanks to advances in deep learning, deep networks can now be used to learn the distribution of normally-exposed images or the real noise distribution, thus improving the visibility of low-light images. The goal of Yufei’s research regarding the low-light enhancement task is in the following ways: 1) prioritize human perceptual quality over some specific metrics in terms of the restored images, 2) enhance from raw images (digital negatives), which contain more information than sRGB images, and 3) close the gap between research and real-world applications.
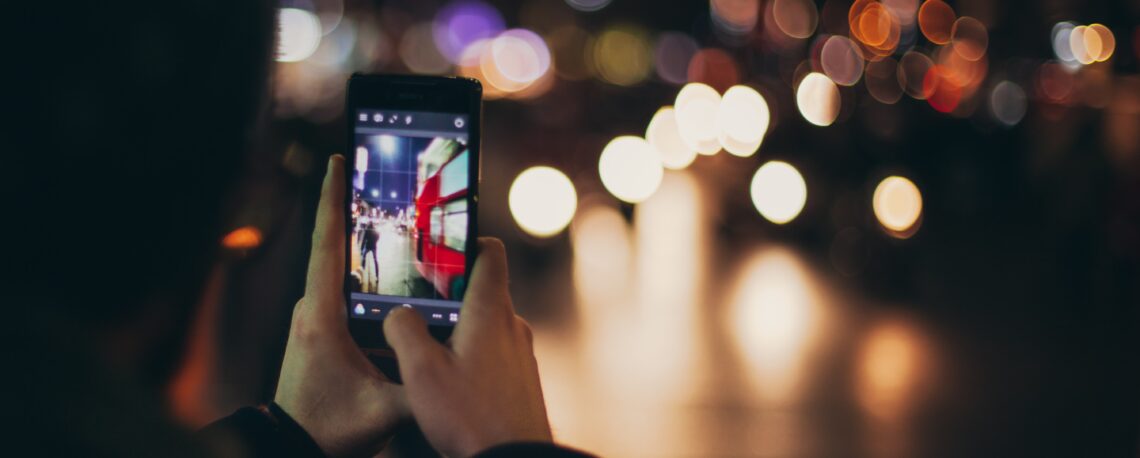