Human action recognition is a fast-developing area with applications ranging from human-computer interaction to video surveillance. According to the types of input data, human action recognition can be grouped into different categories, such as RGB-based, depth-based and 3D skeleton-based. Among these types of inputs, 3D skeleton data, which represents a human body by the locations of body joints in the 3D space, has attracted increasing attention in recent years. In comparison with RGB videos and depth data, 3D skeleton data encodes high-level representations of human behaviours, and is generally lightweight and robust to the appearance variations, surrounding distractions and viewpoint changes, to name a few. Recently, deep neural networks (DNNs) have achieved remarkable success in 3D skeleton-based action recognition. Despite a wide range of impressive results, in order to achieve good performance, current DNN based methods require massive amounts of accurately annotated training data. However, collecting and labelling large-scale training datasets is time-consuming and costly, especially in action recognition that requires sequential-based ground truth labelling. To ease the need for a massive amount of labeled training data, Siyuan’s research focuses on the fewer label issue on human action recognition, including the weakly-supervised, self-supervised representation learning and few-shot learning on skeleton action recognition. Click on the video below to view a presentation on the research project!
Video Player 00:00 00:00 |
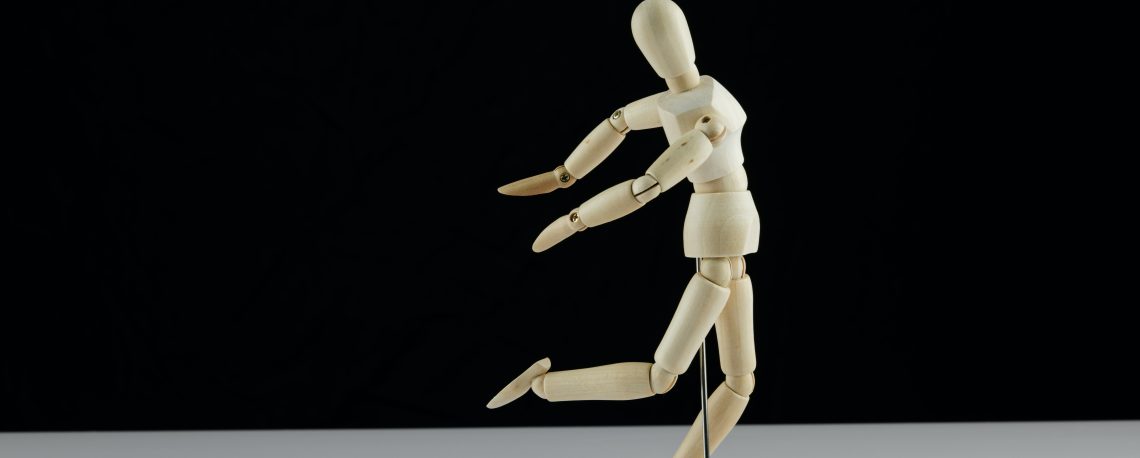