Automatic translation technologies, such as Google Translate, are benefiting billions of people from different cultures around the world by breaking the language barriers between cultures and communities. It reduces the need for human translators and cost per translation. In the context of multi-lingual and multi-cultural environments like Singapore and other Southeast Asian countries, the importance of MT is even more critical as it helps facilitate trades and exchange of ideas. These technologies are powered by Neural Machine Translation (NMT), which is a neural network trained by deep learning to translate texts from one language to another. The core objective of Xuan Phi’s research has been to advance and improve state-of-the-art NMT systems not only in the traditional supervised setup, but also in unsupervised and semi-supervised scenarios, as well as for low-resource languages, such as Malay, Nepali, Tamil, etc. More specifically, his research tackles various existing challenges in NMT, such as suboptimal performance in high-resource languages, significant underperformance and failure in unsupervised machine translation on low-resource languages. Through multiple publications, his proposed methods advance the field by achieving the state of the art in both supervised and unsupervised machine translation. Click on the video below to view a presentation on the research project!
Video Player 00:00 00:00 |
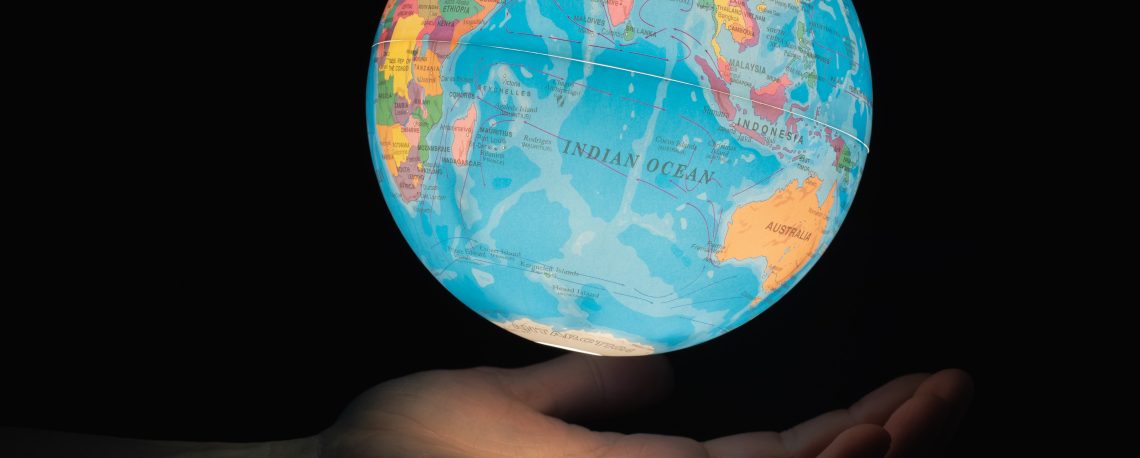