Recommendation explanations, which would make the recommendations more persuasive and acceptable to users, could be generated via either a separated explanation model (post-hoc) upon a base recommendation model as a pipeline approach or an integrated explainable recommendation model. In this thesis, Trung Hoang encompasses both integrated explainable recommendation and post-hoc explanation approaches. Depending on the characteristics of the models, information used, as well as the desired experience that the system wants users to have, the research can produce different forms of recommendation explanations. It could be generic, as they explain how the recommendation engine works, evaluative, accessing the quality of a product and of itself, or comparative, accessing a recommendation in comparison to another reference product. For post-hoc explanation, he proposed Synthesizing Explanation for Explainable Recommendation, which selects sentences (opinion phrases could be substituted to match user preference) from other reviewers satisfying an aspect demand of interests from the target user. For comparative explanation, he proposed to anchor reference products on the previously adopted products in a user history. Experiments on Amazon Reviews dataset show the efficacies of the proposed methods. Click on the video below to view a presentation on the research project!
Video Player 00:00 00:00 |
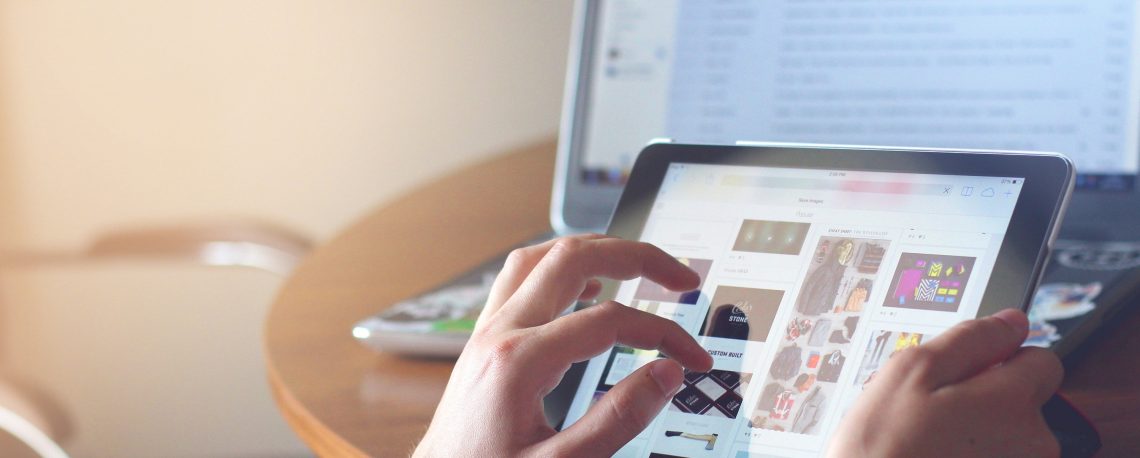